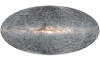
Comprobar eficacia, código CNN.
Python
Publicado el 6 de Marzo del 2024 por Hilario (122 códigos)
158 visualizaciones desde el 6 de Marzo del 2024
IMAGEN A PREDECIR.
-----------------------------
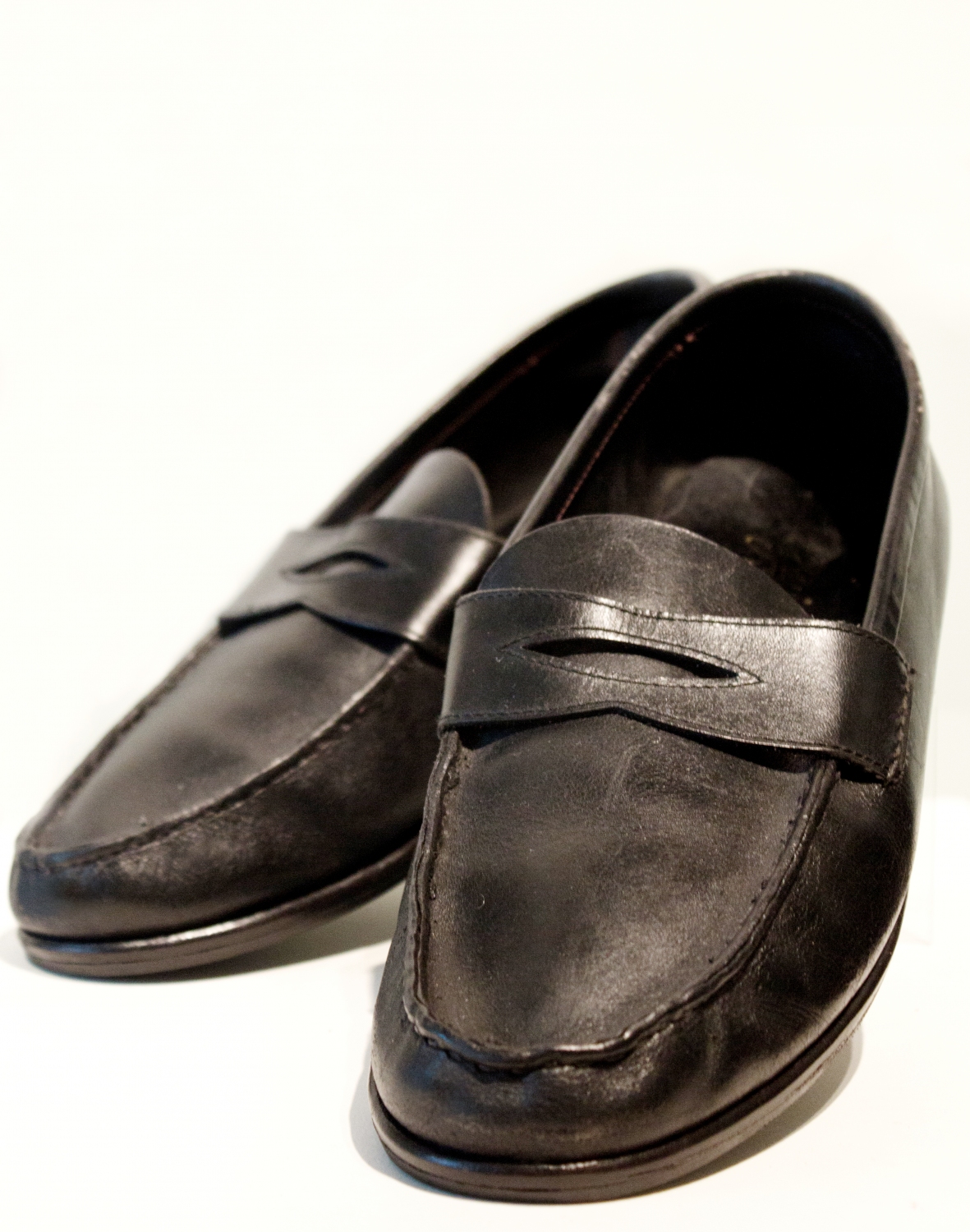
El codigo de estudio, fue recogido en la página oficial de Tensorflow, con el Copyright (c) 2017 de François Chollet.
Ver nota final de uso.
Lo que trato con este ejercicio, es añadirle un tramo de código, con el fin de probar su eficacia al añadirle la predicción de una imagen aportada por mí.
Por lo que pude apreciar, los resultados no son del todo halagueños.
# Copyright (c) 2017 François Chollet
# Permission is hereby granted, free of charge, to any person obtaining a
# copy of this software and associated documentation files (the "Software"),
# to deal in the Software without restriction, including without limitation
# the rights to use, copy, modify, merge, publish, distribute, sublicense,
# and/or sell copies of the Software, and to permit persons to whom the
# Software is furnished to do so, subject to the following conditions:
#
# The above copyright notice and this permission notice shall be included in
# all copies or substantial portions of the Software.
#
# THE SOFTWARE IS PROVIDED "AS IS", WITHOUT WARRANTY OF ANY KIND, EXPRESS OR
# IMPLIED, INCLUDING BUT NOT LIMITED TO THE WARRANTIES OF MERCHANTABILITY,
# FITNESS FOR A PARTICULAR PURPOSE AND NONINFRINGEMENT. IN NO EVENT SHALL
# THE AUTHORS OR COPYRIGHT HOLDERS BE LIABLE FOR ANY CLAIM, DAMAGES OR OTHER
# LIABILITY, WHETHER IN AN ACTION OF CONTRACT, TORT OR OTHERWISE, ARISING
# FROM, OUT OF OR IN CONNECTION WITH THE SOFTWARE OR THE USE OR OTHER
# DEALINGS IN THE SOFTWARE.
-----------------------------
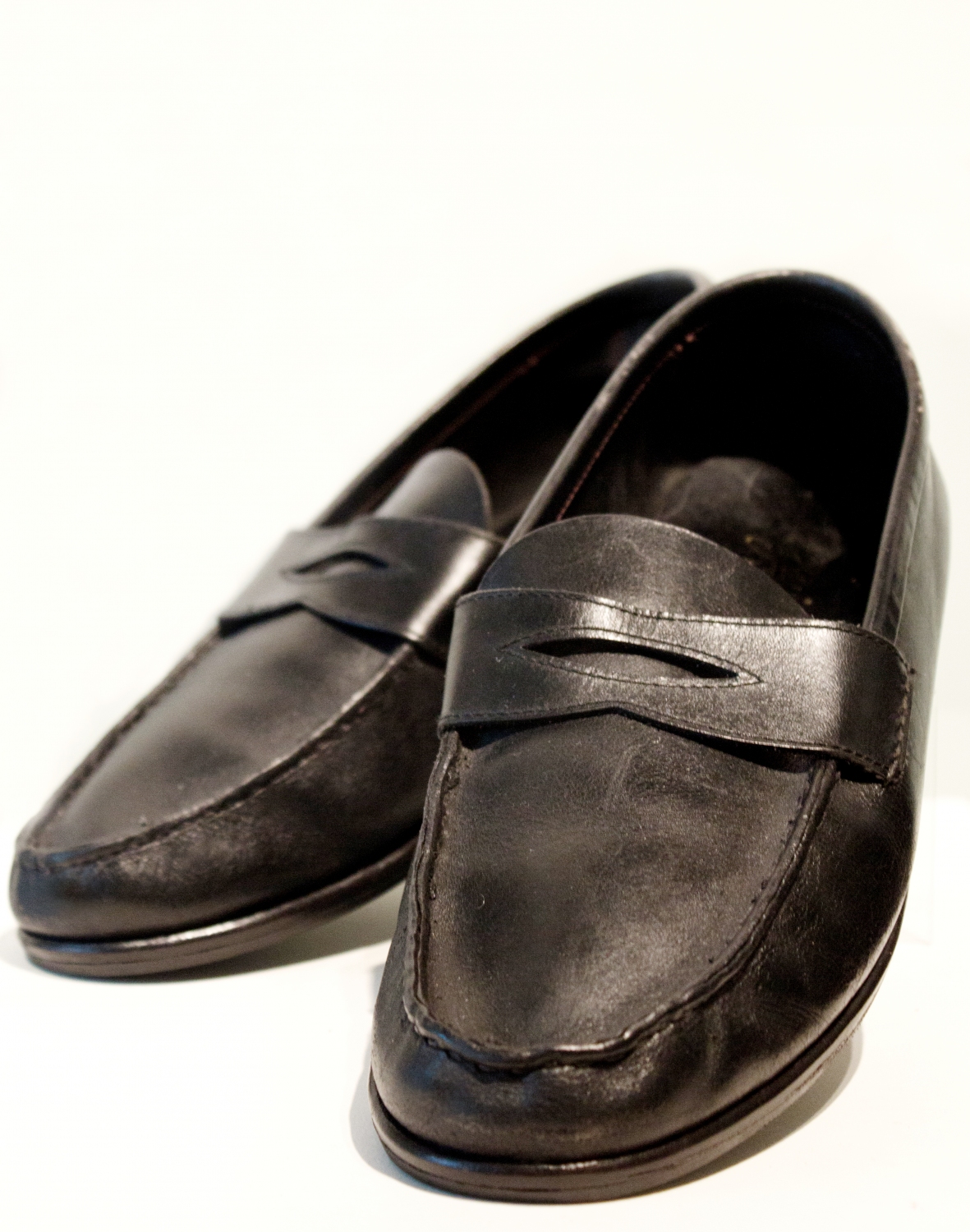
El codigo de estudio, fue recogido en la página oficial de Tensorflow, con el Copyright (c) 2017 de François Chollet.
Ver nota final de uso.
Lo que trato con este ejercicio, es añadirle un tramo de código, con el fin de probar su eficacia al añadirle la predicción de una imagen aportada por mí.
Por lo que pude apreciar, los resultados no son del todo halagueños.
# Copyright (c) 2017 François Chollet
# Permission is hereby granted, free of charge, to any person obtaining a
# copy of this software and associated documentation files (the "Software"),
# to deal in the Software without restriction, including without limitation
# the rights to use, copy, modify, merge, publish, distribute, sublicense,
# and/or sell copies of the Software, and to permit persons to whom the
# Software is furnished to do so, subject to the following conditions:
#
# The above copyright notice and this permission notice shall be included in
# all copies or substantial portions of the Software.
#
# THE SOFTWARE IS PROVIDED "AS IS", WITHOUT WARRANTY OF ANY KIND, EXPRESS OR
# IMPLIED, INCLUDING BUT NOT LIMITED TO THE WARRANTIES OF MERCHANTABILITY,
# FITNESS FOR A PARTICULAR PURPOSE AND NONINFRINGEMENT. IN NO EVENT SHALL
# THE AUTHORS OR COPYRIGHT HOLDERS BE LIABLE FOR ANY CLAIM, DAMAGES OR OTHER
# LIABILITY, WHETHER IN AN ACTION OF CONTRACT, TORT OR OTHERWISE, ARISING
# FROM, OUT OF OR IN CONNECTION WITH THE SOFTWARE OR THE USE OR OTHER
# DEALINGS IN THE SOFTWARE.
Comentarios sobre la versión: V.O. (0)
No hay comentarios